Using an AI to Validate Another AI’s Output
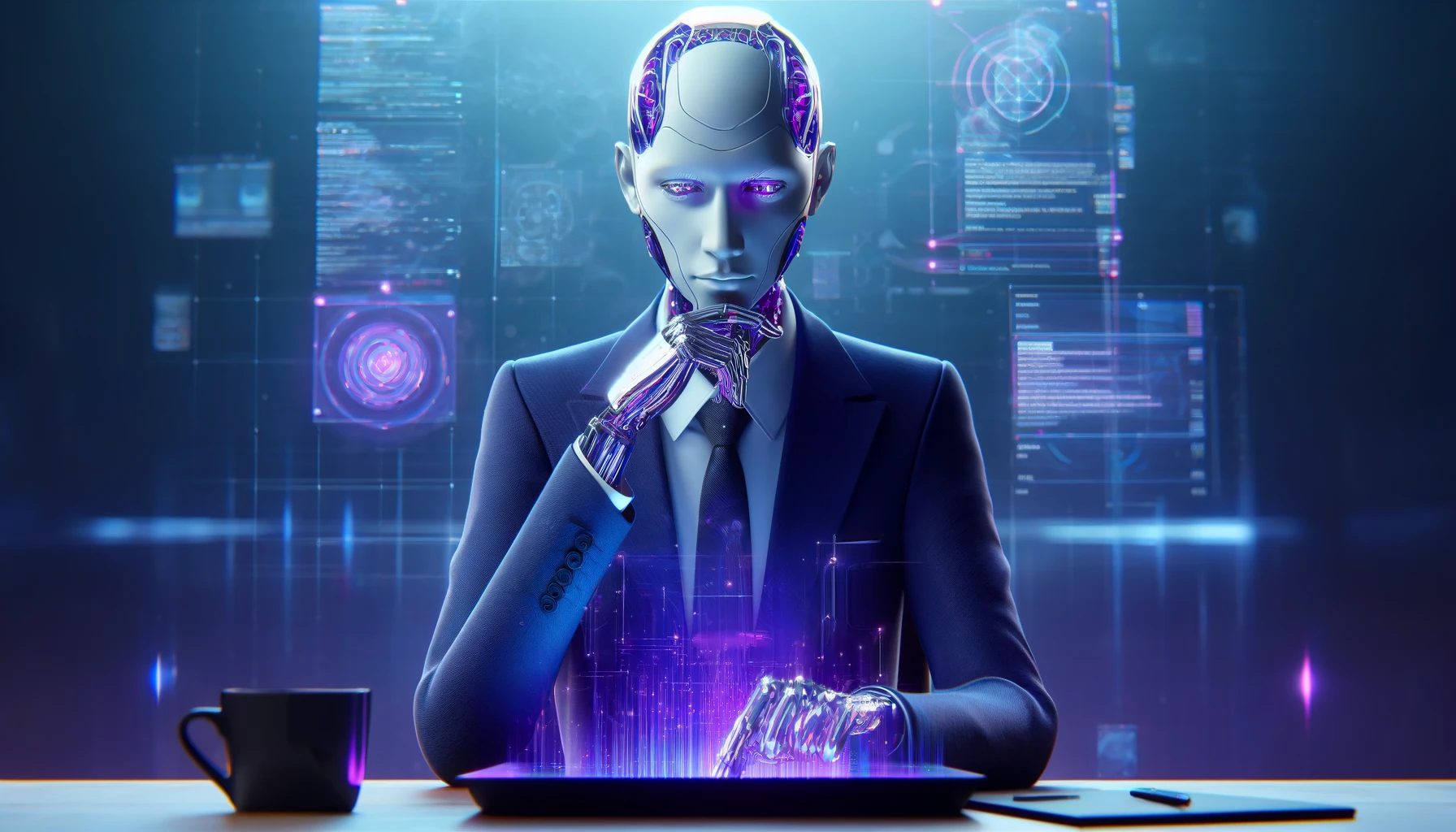
Generative AI can be unreliable at times. Use this shortcut to quickly validate the quality of AI outputs
Have you ever asked ChatGPT the same question twice?
Did you get the same answer?
The answer’s probably no, assuming the question wasn’t too basic.
This is because outputs from generative AI are “non-deterministic”. In other words, answers will vary, which means we need some way to assess the quality of any given output.
There are frameworks available for evaluating AI outputs, but they frequently require a great deal of industry-specific knowledge.
Or you can do what we did at AiSDR, which is take a shortcut and use one generative AI model to check the output from a different model.
TLDR:
- The goal: Confirm whether an AI’s output is sufficiently good
- The tactic: Use a different AI to check the output of the original AI (i.e. use Gemini to check ChatGPT’s output)
- The result: Speed up the process of checking AI outputs
Step 1: Generate an Output
Let’s say you want to take a closer look at the outputs of GPT-4o.
Before you can test an output’s quality, you’ll need to create an output, which can be text, image, audio, or any other type of content.
Step 2: Reformat the Output
Depending on the AI models you use, you might have to “reformat” the output so that the second AI can work with it better. This is especially true if you’re using LLMs from different providers, like Anthropic and OpenAI.
This step is more commonly known as preprocessing.
Common examples of preprocessing include:
- Adding or removing text elements like HTML tags
- Breaking larger texts into smaller parts (i.e. essays into individual paragraphs or paragraphs into individual sentences)
- Identifying people, organizations, locations, or dates
- Converting data into a format that’s compatible with the second model
Step 3: Evaluate the Output
Once the output’s been preprocessed, you can enter the output into the second AI (e.g. Claude 3.5 Sonnet). To systematize your evaluation, you should create a set of criteria by which you can compare AI models.
The set of criteria you create will vary by situation, but common criteria include:
- Computational efficiency – Is the model efficient in terms of resource usage, speed, scalability, and performance?
- Currency – Is the content reflective of new information?
- Relevancy – Is the content on topic?
- Authority – Is the content backed by reputable sources or evidence?
- Accuracy – Is the content accurate and free of errors or misleading information?
- Purpose – Is the content in line with the intended purpose?
You can then use the results to determine whether or not the original AI is effective and suitable for your requirements, which could be anything from model distillation to carrying out simple day-to-day work tasks.
The Result
Using a second AI to validate an AI has helped us achieve several good results:
Resource efficiency – AI’s capacity to process large amounts of data faster and more thoroughly than humans has helped us allocate people and resources to strategic-level tasks. It’s also allowed us to quickly fine-tune our AI’s ability to flag potential issues and non-standard sales emails, as well as recognize common types of messages like auto-replies.
- How you can use it – Delegate high-volume, low-level tasks that can be completed with minimal training to AI. Tell the AI when it makes a mistake, and provide examples of what you expect. The more AI works with data, the better its performance. It’s not much different than onboarding a new teammate.
Scalability – Our AI works with thousands of emails each day. If our team was stuck manually checking and classifying every email, we wouldn’t have much time to focus on product development.
- How you can use this – If you’re uncertain about how effective AI is at classification or other high-volume, low-level tasks, you can use a different AI to test its ability. This will help you build confidence in your AI or provide insight into where improvements are needed.
Redundancy – Learning how to interact with different AI models has helped us prepare for any situation when a major AI model like ChatGPT goes down. If this happens, we can simply switch the AI engine as we developed AiSDR to be language agnostic.
- How you can use this – Design your processes and product development to also be language agnostic. This limits your exposure to an AI going down as your processes will continue to operate as expected.
However, there is one caveat to using generative AI.
Specifically, you’ll have to get used to and work around AI behaving unpredictably.